일 | 월 | 화 | 수 | 목 | 금 | 토 |
---|---|---|---|---|---|---|
1 | 2 | 3 | 4 | 5 | 6 | |
7 | 8 | 9 | 10 | 11 | 12 | 13 |
14 | 15 | 16 | 17 | 18 | 19 | 20 |
21 | 22 | 23 | 24 | 25 | 26 | 27 |
28 | 29 | 30 |
- 하둡
- HIVE
- 빅데이터처리
- ggplot
- word2vec
- pandas
- SQL
- Python
- R그래프
- lstm
- NLP
- 그래프시각화
- CNN
- 데이터시각화
- 데이터
- r
- Deeplearning
- 데이터분석
- R프로그래밍
- AI
- 기계학습
- 그래프
- 딥러닝
- 빅데이터
- 머신러닝
- 데이터처리
- 자연어처리
- R시각화
- 주가예측
- Hadoop
- Today
- Total
목록자연어처리 (3)
욱이의 냉철한 공부
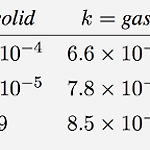
* 자료출저 및 참고논문 - 논문 GloVe : 2014, Global Vectors for Word Representation * Word Representation 분류체계 1. Discrete Representation : Local Representation 1) One - hot Vector - One - hot Vector 2) Count Based - Bag of Words (BoW) - Document-Term Matrix (DTM) - (TDM) - Term Frequency-Inverse Document Frequency (TF - IDF) - N-gram Language Model (N-gram) 2. Continuous Representation 1) Prediction Base..
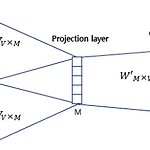
* 자료출저 및 참고논문 - 강의 Coursera, Andrew Ng 교수님 인터넷 강의 - 논문 Word2Vec: 2013, Effcient Estimation of Word Representations in Vector Space * Word Representation 관점 : Word Embedding 만들기 1. Discrete Representation : Local Representation 1) One - hot Vector - One - hot Vector 2) Count Based - Bag of Words (BoW) - Document-Term Matrix (DTM) - (TDM) - Term Frequency-Inverse Document Frequency (TF - IDF) - N-..
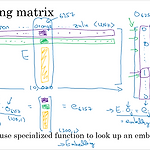
* 자료출저 및 참고논문 - 강의 Coursera, Andrew Ng 교수님 인터넷 강의 - 논문 NPLM : A Neural Probabilistic Language Model * Word Representation 분류체계 1. Discrete Representation : Local Representation 1) One - hot Vector - One - hot Vector 2) Count Based - Bag of Words (BoW) - Document-Term Matrix (DTM) - (TDM) - Term Frequency-Inverse Document Frequency (TF - IDF) - N-gram Language Model (N-gram) 2. Continuous Repre..